Aim and Scope
Series of Artificial Intelligence and Machine Learning is an online, peer-reviewed journal that serves as a prominent platform for the publication of articles related to the fields of computer science, artificial intelligence, and machine learning. We are committed to advancing knowledge in these dynamic domains by providing easy and globalized access to academic scholars and researchers through open-access publication.
Our Mission:
Our primary mission is to present reliable and cutting-edge information in the fields of computer science, artificial intelligence, and machine learning. We strive to foster intellectual exchange, facilitate collaboration, and promote the dissemination of high-quality research and insights.
Key Features:
- We pride ourselves on publishing high-quality peer-reviewed articles, ensuring that our readers have access to the latest advancements and breakthroughs in AI and ML.
- All scientific journals published by us, including “Series of Artificial Intelligence and Machine Learning,” adhere to the policies of the Creative Commons Attribution License. This grants permission for copying, distributing, and adapting any work from our open-access journals, provided that the original source and work are correctly attributed.
Open Access and Immediate Accessibility:
We are dedicated to open-access principles, making all the articles available for reading and downloading purposes immediately after they are published. Our commitment to open access ensures that the knowledge shared in our journal reaches a global audience without barriers.
By publishing in “Series of Artificial Intelligence and Machine Learning,” you become part of a vibrant community of AI and ML enthusiasts and researchers, contributing to the growth of these transformative technologies.
Journal Highlights
Topics covered in Series of Artificial Intelligence and Machine Learning are not limited to:
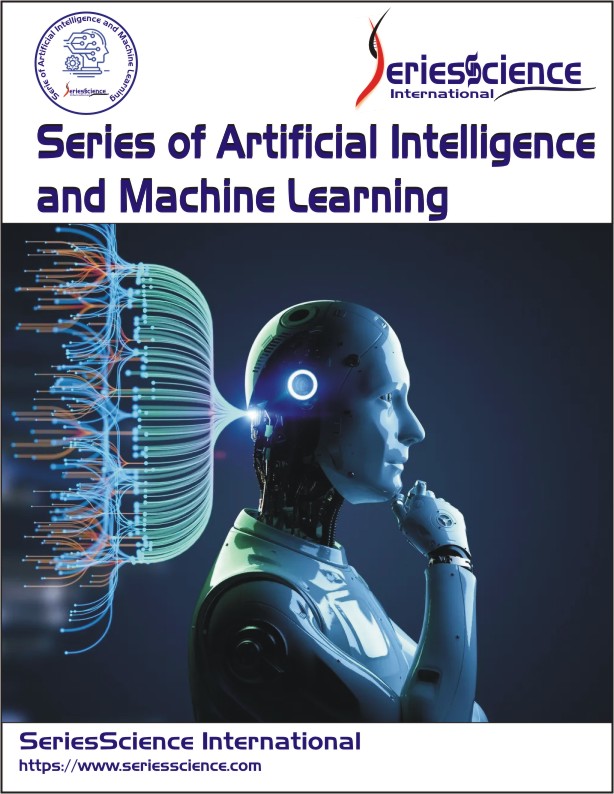
Journal Information
Journal Name : Series of Artificial Intelligence and Machine Learning
Journal Short Name : Series Artificial Intel Machine Learn.
Journal Code : #JSAIML
DOI : 10.54178
Publication Model : Open Access
Peer Review Model : Double Blind